Lineage Prevalence Analysis
The outbreak_data
package has contains multiple endpoints that can collect information on SARS-CoV-2 lineages. Pulling data from a combination of endpoints will allow you to conduct your own analysis on the progression of SARS-CoV-2. On this page, you’ll find a few example workflows that demonstrate how to collect, manipulate, and visualize prevalence data in SARS-CoV-2 lineages.
Here is how we would go about collecting data to find all the XBB lineages prevalent in India within a 1-year timeframe:
# Perform authentication if you haven't already
from outbreak_data import authenticate_user
authenticate_user.authenticate_new_user()
# Import outbreak_data package
from outbreak_data import outbreak_data as od
# Get the prevalence of all circulating XBB lineages in India
data = od.prevalence_by_location("IND", startswith = 'xbb')
# multiply prevalence values by 100% for scale
data['prevalence_rolling'] = data['prevalence_rolling'].apply(lambda x: x*100)
# Search for data based on date range
data = data.sort_values(by="date")
data = data.loc[data["date"].between("2020-09-12", "2022-03-31")]
## Use the visual package of your choice to create an area graph using your data
import altair as alt
# Graph of results
alt.Chart(data, title = "Lineage Prevalence in India").mark_area().encode(
x='date:T',
y=alt.Y('prevalence_rolling:Q'),
color = 'lineage:N')
date total_count lineage_count lineage prevalence \
3014 2022-09-12 0 0 xbb.1.16 0.000000
3781 2022-09-12 0 0 xbb.2.3 0.000000
2593 2022-09-12 152 2 xbb.1 0.013158
3782 2022-09-13 0 0 xbb.2.3 0.000000
3015 2022-09-13 0 0 xbb.1.16 0.000000
... ... ... ... ... ...
4086 2023-03-31 196 2 xbb.2.3.2 0.010204
3322 2023-03-31 196 29 xbb.1.16.1 0.147959
2793 2023-03-31 196 1 xbb.1 0.005102
3381 2023-03-31 196 7 xbb.1.16.2 0.035714
3981 2023-03-31 196 15 xbb.2.3 0.076531
prevalence_rolling
3014 0.000000
3781 0.000000
2593 0.003451
3782 0.000000
3015 0.000000
... ...
4086 0.031184
3322 0.144578
2793 0.014174
3381 0.045358
3981 0.084337
[985 rows x 6 columns]
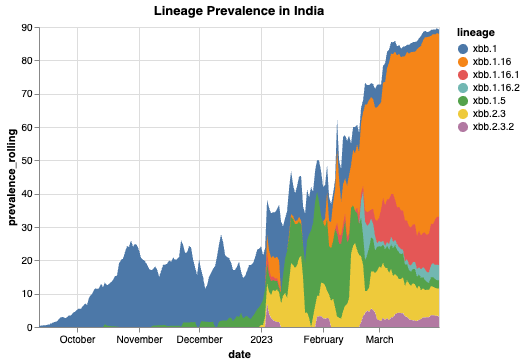
Note
The Vega-Altair visualization package is used for demonstration purposes. However, any Python visual package can be used to create graphical representations of the data.
Finding the Most Prevalent Lineages
If we wanted to determine and plot the top four most prevalent lineages in India, we can make a few queries and use a few simple commands to create a table that shows us what these lineages are:
data=od.prevalence_by_location("IND")
most_prev = data.groupby('lineage').apply(max) # Finds the lineages with the most hits
most_prev = most_prev.mask(most_prev == '').dropna(how = 'any') # Drop any unknowns
most_prev = most_prev.iloc[:4]
print(most_prev)
date total_count lineage_count lineage prevalence \
lineage
ba.2 2023-04-20 5668 1445 ba.2 0.822785
ba.2.10.1 2023-04-19 5668 93 ba.2.10.1 0.285714
bq.1.1 2023-03-27 402 7 bq.1.1 0.428571
ch.1.1 2023-02-13 119 4 ch.1.1 0.400000
prevalence_rolling
lineage
ba.2 0.677541
ba.2.10.1 0.095541
bq.1.1 0.156863
ch.1.1 0.066667
Next we’ll collect the prevalence data on each of the four lineages:
# Retrieve the official data on the prevalences of these lineages using `daily_prev()`
d1 = od.daily_prev('ba.2', "IND")
d2 = od.daily_prev('ba.2.10.1', "IND")
d3 = od.daily_prev('bq.1.1', "IND")
d4 = od.daily_prev( 'ch.1.1', "IND")
# Formatting for creating the graph
d1['lineage'] = 'ba.2'
d2['lineage'] = 'ba.2.10.1'
d3['lineage'] = 'bq.1.1'
d4['lineage'] = 'ch.1.1'
# Group together data from each lineage
data = pd.concat([d1, d2, d3, d4])
data = data.rename(columns = {'proportion': 'proportion (%)'})
#Pick a date range to analyze
data = data.sort_values(by="date")
data = data.loc[data["date"].between("2022-09-12", "2023-03-31")]
# Increase prevalence by 100%
data['proportion'] = data['proportion'].apply(lambda x: x*100)
#Graph using preferred visual package
import altair as alt
alt.Chart(data, title = "Top 4 Most Prevalent Lineages in India").mark_area().encode(
x='date:T',
y=alt.Y('proportion (%):Q'),
color = 'lineage:N')
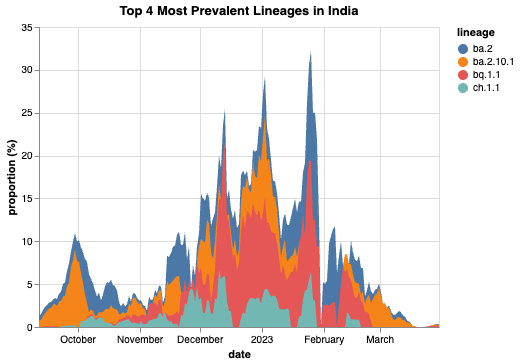